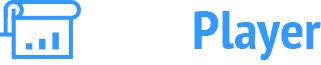
- My presentations

Auth with social network:
Download presentation
We think you have liked this presentation. If you wish to download it, please recommend it to your friends in any social system. Share buttons are a little bit lower. Thank you!
Presentation is loading. Please wait.
PROCESSING, ANALYSIS & INTERPRETATION OF DATA
Published by Maurice Lamb Modified over 9 years ago
Similar presentations
Presentation on theme: "PROCESSING, ANALYSIS & INTERPRETATION OF DATA"— Presentation transcript:
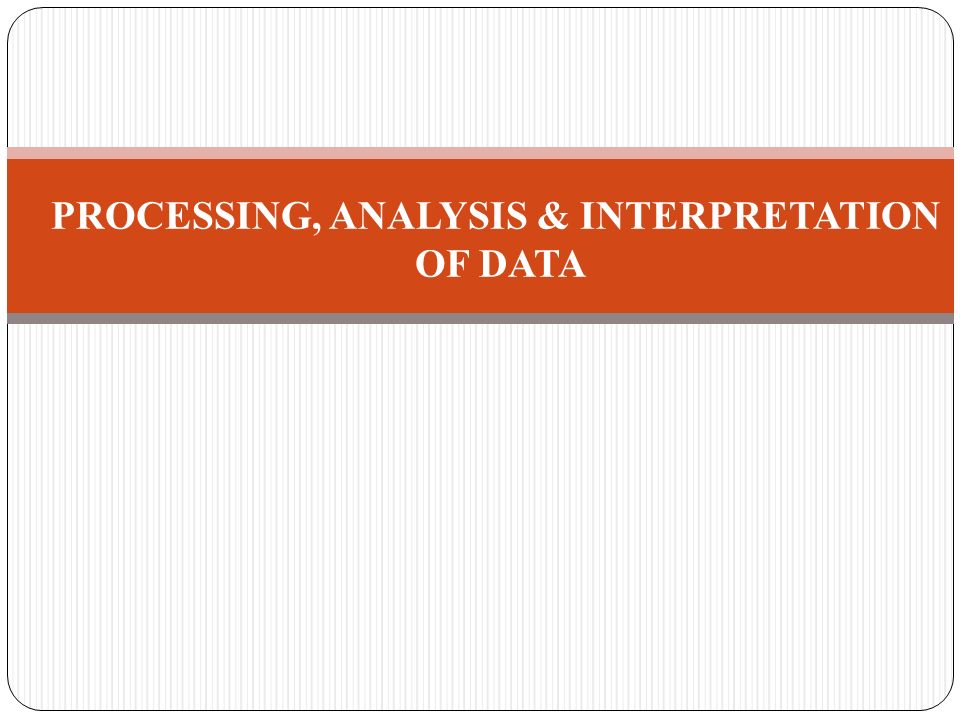
Preparing Data for Quantitative Analysis
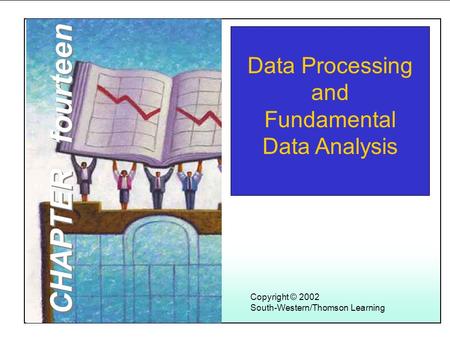
Learning Objectives Copyright © 2002 South-Western/Thomson Learning Data Processing and Fundamental Data Analysis CHAPTER fourteen.
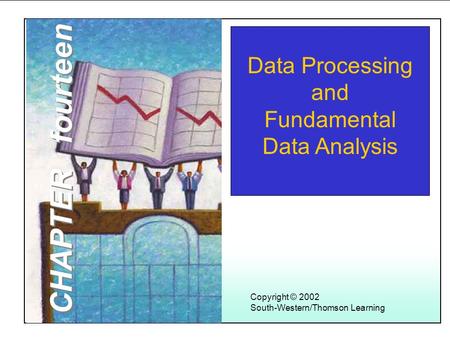
Learning Objectives 1 Copyright © 2002 South-Western/Thomson Learning Data Processing and Fundamental Data Analysis CHAPTER fourteen.
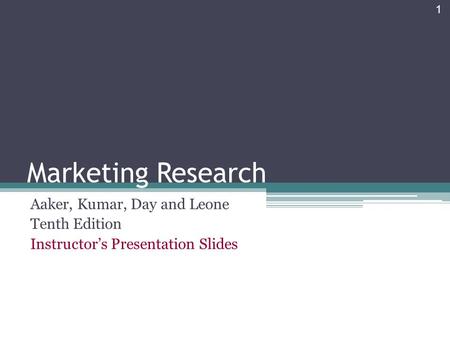
Marketing Research Aaker, Kumar, Day and Leone Tenth Edition Instructor’s Presentation Slides 1.

1 QUANTITATIVE DESIGN AND ANALYSIS MARK 2048 Instructor: Armand Gervais
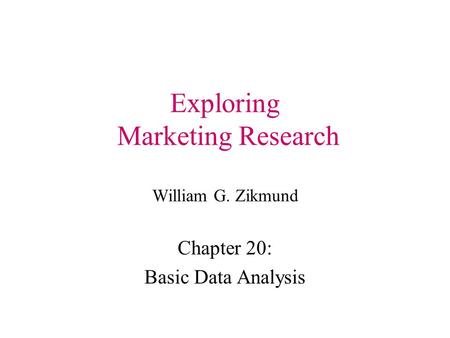
Exploring Marketing Research William G. Zikmund Chapter 20: Basic Data Analysis.
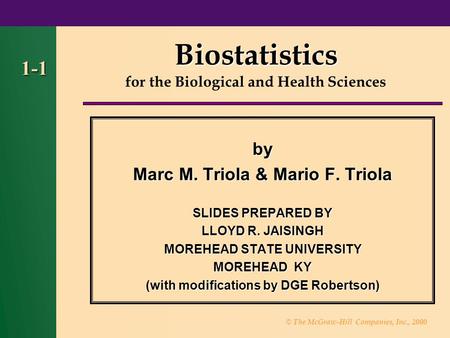
© The McGraw-Hill Companies, Inc., by Marc M. Triola & Mario F. Triola SLIDES PREPARED BY LLOYD R. JAISINGH MOREHEAD STATE UNIVERSITY MOREHEAD.
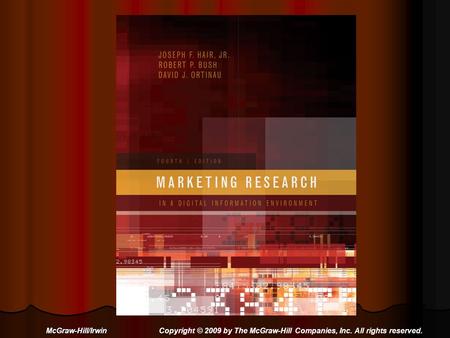
McGraw-Hill/Irwin McGraw-Hill/Irwin Copyright © 2009 by The McGraw-Hill Companies, Inc. All rights reserved.
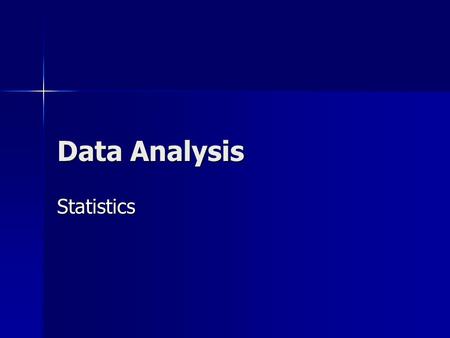
Data Analysis Statistics. OVERVIEW Getting Ready for Data Collection Getting Ready for Data Collection The Data Collection Process The Data Collection.
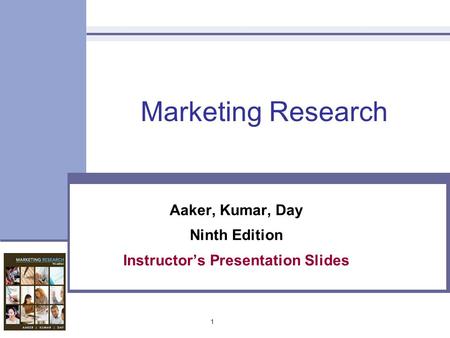
Aaker, Kumar, Day Ninth Edition Instructor’s Presentation Slides
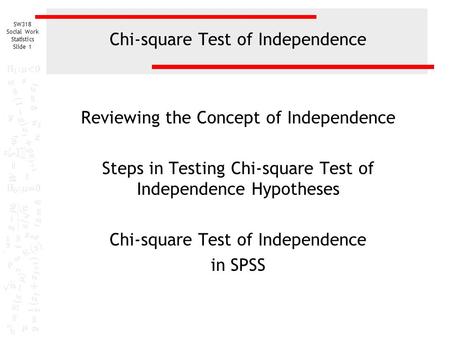
Chi-square Test of Independence
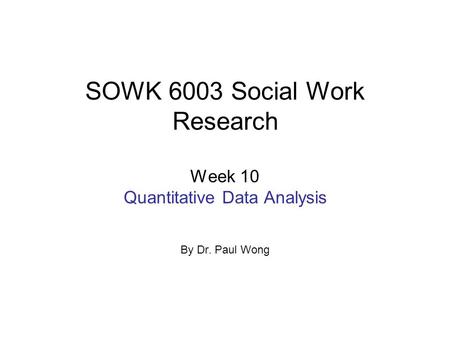
SOWK 6003 Social Work Research Week 10 Quantitative Data Analysis
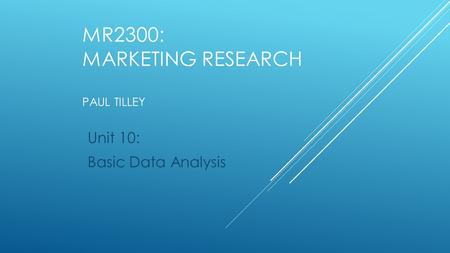
MR2300: MARKETING RESEARCH PAUL TILLEY Unit 10: Basic Data Analysis.
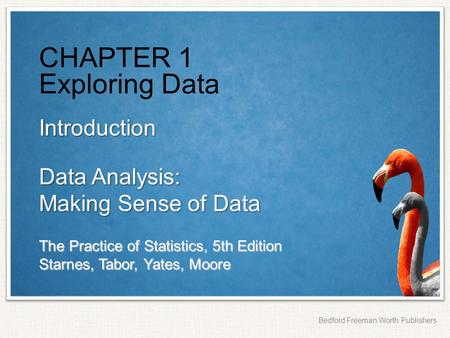
CHAPTER 1 Exploring Data
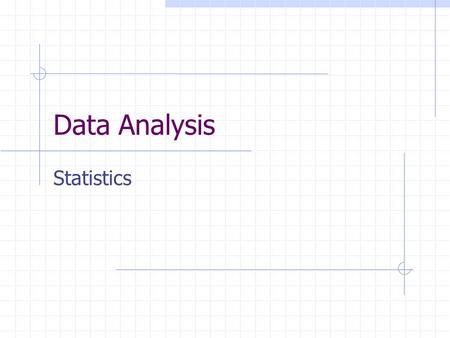
Data Analysis Statistics. OVERVIEW Getting Ready for Data Collection The Data Collection Process Getting Ready for Data Analysis Descriptive Statistics.
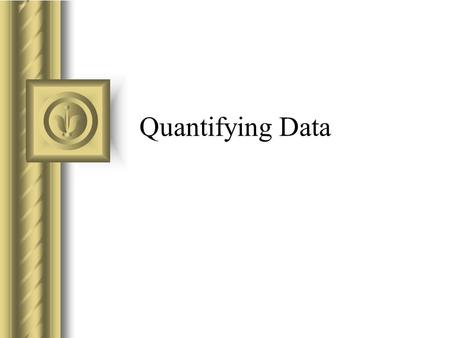
Quantifying Data.
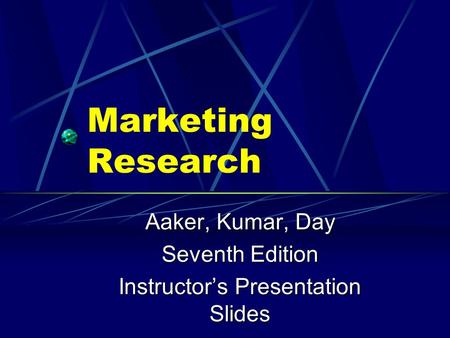
Marketing Research Aaker, Kumar, Day Seventh Edition Instructor’s Presentation Slides.
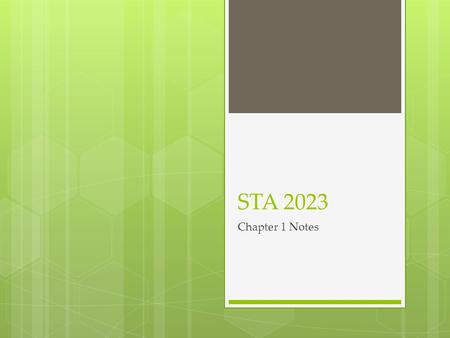
STA 2023 Chapter 1 Notes. Terminology Data: consists of information coming from observations, counts, measurements, or responses. Statistics: the.
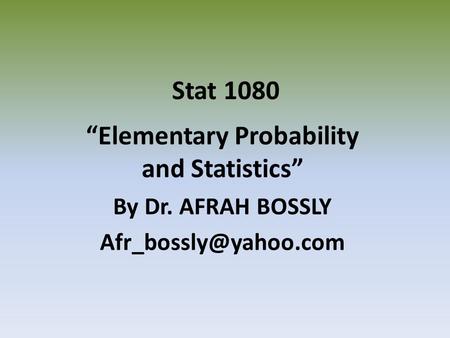
Stat 1080 “Elementary Probability and Statistics” By Dr. AFRAH BOSSLY
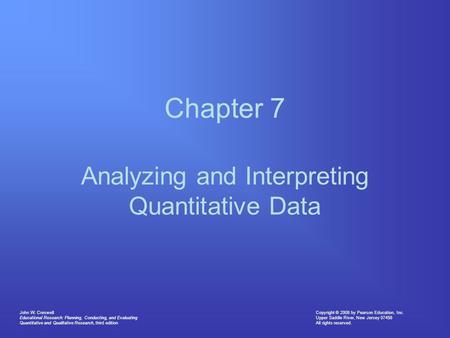
Copyright © 2008 by Pearson Education, Inc. Upper Saddle River, New Jersey All rights reserved. John W. Creswell Educational Research: Planning,
About project
© 2024 SlidePlayer.com Inc. All rights reserved.
- Privacy Policy

Home » Data Analysis – Process, Methods and Types
Data Analysis – Process, Methods and Types
Table of Contents
Data analysis is the systematic process of inspecting, cleaning, transforming, and modeling data to uncover meaningful insights, support decision-making, and solve specific problems. In today’s data-driven world, data analysis is crucial for businesses, researchers, and policymakers to interpret trends, predict outcomes, and make informed decisions. This article delves into the data analysis process, commonly used methods, and the different types of data analysis.

Data Analysis
Data analysis involves the application of statistical, mathematical, and computational techniques to make sense of raw data. It transforms unorganized data into actionable information, often through visualizations, statistical summaries, or predictive models.
For example, analyzing sales data over time can help a retailer understand seasonal trends and forecast future demand.
Importance of Data Analysis
- Informed Decision-Making: Helps stakeholders make evidence-based choices.
- Problem Solving: Identifies patterns, relationships, and anomalies in data.
- Efficiency Improvement: Optimizes processes and operations through insights.
- Strategic Planning: Assists in setting realistic goals and forecasting outcomes.
Data Analysis Process
The process of data analysis typically follows a structured approach to ensure accuracy and reliability.
1. Define Objectives
Clearly articulate the research question or business problem you aim to address.
- Example: A company wants to analyze customer satisfaction to improve its services.
2. Data Collection
Gather relevant data from various sources, such as surveys, databases, or APIs.
- Example: Collect customer feedback through online surveys and customer service logs.
3. Data Cleaning
Prepare the data for analysis by removing errors, duplicates, and inconsistencies.
- Example: Handle missing values, correct typos, and standardize formats.
4. Data Exploration
Perform exploratory data analysis (EDA) to understand data patterns, distributions, and relationships.
- Example: Use summary statistics and visualizations like histograms or scatter plots.
5. Data Transformation
Transform raw data into a usable format by scaling, encoding, or aggregating.
- Example: Convert categorical data into numerical values for machine learning algorithms.
6. Analysis and Interpretation
Apply appropriate methods or models to analyze the data and extract insights.
- Example: Use regression analysis to predict customer churn rates.
7. Reporting and Visualization
Present findings in a clear and actionable format using dashboards, charts, or reports.
- Example: Create a dashboard summarizing customer satisfaction scores by region.
8. Decision-Making and Implementation
Use the insights to make recommendations or implement strategies.
- Example: Launch targeted marketing campaigns based on customer preferences.
Methods of Data Analysis
1. statistical methods.
- Descriptive Statistics: Summarizes data using measures like mean, median, and standard deviation.
- Inferential Statistics: Draws conclusions or predictions from sample data using techniques like hypothesis testing or confidence intervals.
2. Data Mining
Data mining involves discovering patterns, correlations, and anomalies in large datasets.
- Example: Identifying purchasing patterns in retail through association rules.
3. Machine Learning
Applies algorithms to build predictive models and automate decision-making.
- Example: Using supervised learning to classify email spam.
4. Text Analysis
Analyzes textual data to extract insights, often used in sentiment analysis or topic modeling.
- Example: Analyzing customer reviews to understand product sentiment.
5. Time-Series Analysis
Focuses on analyzing data points collected over time to identify trends and patterns.
- Example: Forecasting stock prices based on historical data.
6. Data Visualization
Transforms data into visual representations like charts, graphs, and heatmaps to make findings comprehensible.
- Example: Using bar charts to compare monthly sales performance.
7. Predictive Analytics
Uses statistical models and machine learning to forecast future outcomes based on historical data.
- Example: Predicting the likelihood of equipment failure in a manufacturing plant.

8. Diagnostic Analysis
Focuses on identifying causes of observed patterns or trends in data.
- Example: Investigating why sales dropped in a particular quarter.
Types of Data Analysis
1. descriptive analysis.
- Purpose: Summarizes raw data to provide insights into past trends and performance.
- Example: Analyzing average customer spending per month.
2. Exploratory Analysis
- Purpose: Identifies patterns, relationships, or hypotheses for further study.
- Example: Exploring correlations between advertising spend and sales.
3. Inferential Analysis
- Purpose: Draws conclusions or makes predictions about a population based on sample data.
- Example: Estimating national voter preferences using survey data.
4. Diagnostic Analysis
- Purpose: Examines the reasons behind observed outcomes or trends.
- Example: Investigating why website traffic decreased after a redesign.
5. Predictive Analysis
- Purpose: Forecasts future outcomes based on historical data.
- Example: Predicting customer churn using machine learning algorithms.
6. Prescriptive Analysis
- Purpose: Recommends actions based on data insights and predictive models.
- Example: Suggesting the best marketing channels to maximize ROI.
Tools for Data Analysis
1. programming languages.
- Python: Popular for data manipulation, analysis, and machine learning (e.g., Pandas, NumPy, Scikit-learn).
- R: Ideal for statistical computing and visualization.
2. Data Visualization Tools
- Tableau: Creates interactive dashboards and visualizations.
- Power BI: Microsoft’s tool for business intelligence and reporting.
3. Statistical Software
- SPSS: Used for statistical analysis in social sciences.
- SAS: Advanced analytics, data management, and predictive modeling tool.
4. Big Data Platforms
- Hadoop: Framework for processing large-scale datasets.
- Apache Spark: Fast data processing engine for big data analytics.
5. Spreadsheet Tools
- Microsoft Excel: Widely used for basic data analysis and visualization.
- Google Sheets: Collaborative online spreadsheet tool.
Challenges in Data Analysis
- Data Quality Issues: Missing, inconsistent, or inaccurate data can compromise results.
- Scalability: Analyzing large datasets requires advanced tools and computing power.
- Bias in Data: Skewed datasets can lead to misleading conclusions.
- Complexity: Choosing the appropriate analysis methods and models can be challenging.
Applications of Data Analysis
- Business: Improving customer experience through sales and marketing analytics.
- Healthcare: Analyzing patient data to improve treatment outcomes.
- Education: Evaluating student performance and designing effective teaching strategies.
- Finance: Detecting fraudulent transactions using predictive models.
- Social Science: Understanding societal trends through demographic analysis.
Data analysis is an essential process for transforming raw data into actionable insights. By understanding the process, methods, and types of data analysis, researchers and professionals can effectively tackle complex problems, uncover trends, and make data-driven decisions. With advancements in tools and technology, the scope and impact of data analysis continue to expand, shaping the future of industries and research.
- McKinney, W. (2017). Python for Data Analysis: Data Wrangling with Pandas, NumPy, and IPython . O’Reilly Media.
- Han, J., Pei, J., & Kamber, M. (2011). Data Mining: Concepts and Techniques . Morgan Kaufmann.
- Provost, F., & Fawcett, T. (2013). Data Science for Business: What You Need to Know About Data Mining and Data-Analytic Thinking . O’Reilly Media.
- Montgomery, D. C., & Runger, G. C. (2018). Applied Statistics and Probability for Engineers . Wiley.
- Tableau Public (2023). Creating Data Visualizations and Dashboards . Retrieved from https://www.tableau.com.
About the author
Muhammad Hassan
Researcher, Academic Writer, Web developer
You may also like

Critical Analysis – Types, Examples and Writing...

Research Questions – Types, Examples and Writing...

Research Problem – Examples, Types and Guide

Research Process – Steps, Examples and Tips

Scope of the Research – Writing Guide and...

References in Research – Types, Examples and...

IMAGES
VIDEO